Unlocking the Power of Data Analytics: An In-Depth Guide
- Purva Yadav
- Jul 23, 2024
- 3 min read
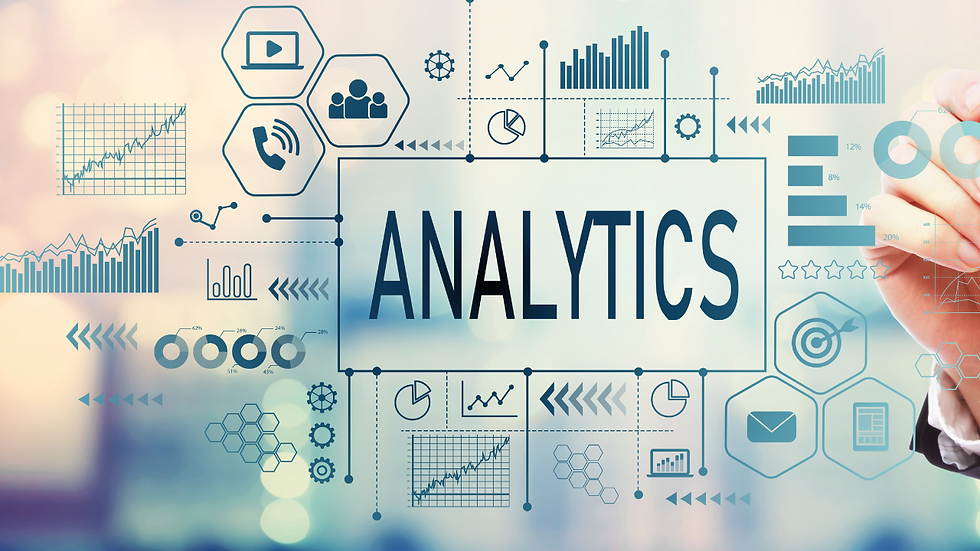
In the modern digital landscape, data analytics has become essential for businesses, enabling them to transform raw data into actionable insights that drive strategic decision-making. This guide explores the fundamentals of data analytics, its significance, tools, techniques, and future trends shaping this dynamic field.
What is Data Analytics?
Data analytics is the process of examining datasets to draw meaningful conclusions. This process includes various techniques such as data mining, which involves extracting valuable information from large datasets, and statistical analysis, which applies statistical tests to identify trends and patterns.
Four primary categories exist for data analytics:
Descriptive Analytics: responds to the query, "What happened?" by summarizing past data.
Diagnostic Analytics: Answers the question, "Why did it happen?" by identifying cause-and-effect relationships in data.
Predictive Analytics: Answers the question, "What could happen?" by utilizing machine learning methods and statistical models.
Prescriptive Analytics: Answers the question, "What should we do?" by suggesting potential outcomes based on data analysis.
The Importance of Data Analytics
Data analytics is vital for several reasons:
Informed Decision-Making: Enables organizations to make data-driven decisions, reducing dependence on intuition.
Operational Efficiency: Helps businesses identify inefficiencies and streamline their operations.
Customer Insights: Provides a deeper understanding of customer behavior, preferences, and trends, facilitating personalized marketing strategies.
Competitive Advantage: Allows organizations to stay ahead of competitors by quickly adapting to market changes and trends.
Risk Management: aids in locating any hazards and putting mitigation strategies in place.
Tools and Techniques in Data Analytics
Several tools and techniques are used to process and analyze data effectively:
Data Collection and Cleaning: Tools like Apache Hadoop and Talend ensure data accuracy and consistency.
Data Visualization: Visualization tools like Tableau and Power BI present data insights through graphs, charts, and dashboards.
Statistical Analysis: Software like R and SAS perform statistical tests and analysis.
Machine Learning: Tools like TensorFlow and scikit-learn build predictive models using machine learning algorithms.
Big Data Processing: Technologies like Apache Spark and Apache Flink efficiently process large volumes of data.
Techniques in Data Analytics
Regression Analysis: Identifies relationships between variables and predicts future outcomes.
Classification: Categorizes data into predefined classes, useful in spam detection and image recognition.
Clustering: Groups similar data points together, commonly used in market segmentation and customer profiling.
Anomaly Detection: Identifies outliers in data, which can indicate fraud or network intrusions.
Natural Language Processing (NLP): Analyzes textual data to extract meaningful information, widely used in sentiment analysis and chatbots.
The Future of Data Analytics
The field of data analytics is continuously evolving, with several emerging trends poised to shape its future:
Artificial Intelligence (AI) and Machine Learning: AI-driven analytics is becoming more prevalent, enabling more accurate and faster insights.
Automated Analytics: Automation tools simplify the analytics process, making it accessible to non-technical users.
Edge Computing: Processes data closer to its source, reducing latency, and improving real-time analytics capabilities.
Enhanced Data Privacy and Security: With increasing data breaches, there is a growing focus on implementing robust security measures to protect data.
Data Democratization: Efforts are being made to make data accessible to all members of an organization, fostering a data-driven culture.
Challenges in Data Analytics
Despite its numerous benefits, data analytics comes with its set of challenges:
Data Quality: Ensuring the accuracy, completeness, and reliability of data is a major challenge.
Data Integration: Integrating data from various sources and formats can be complex and time-consuming.
Scalability: Handling and processing large volumes of data efficiently remains a critical issue.
Skill Gap: There is a significant demand for skilled data analysts, and the shortage of talent can hinder analytics initiatives.
Cost: Implementing data analytics solutions can be costly, especially for small and medium-sized enterprises.
Conclusion
Data analytics is a powerful tool that enables businesses to unlock the full potential of their data. By leveraging the right tools and techniques, organizations can gain valuable insights, improve decision-making, and stay competitive in the rapidly changing market landscape. As technology advances, the future of data analytics looks promising, with innovations such as AI and automation set to further revolutionize the field. However, businesses must also be mindful of the challenges and invest in quality data management and skilled professionals to fully reap the benefits of data analytics. For those looking to enhance their skills, taking a Data Analytics course in Dehradun, Delhi, Noida, Agra and other cities of India can provide a solid foundation and practical knowledge to excel in this field.
Comments