Understanding Data Science: Its Evolution, Components, and Impact
- Purva Yadav
- Sep 11, 2024
- 3 min read
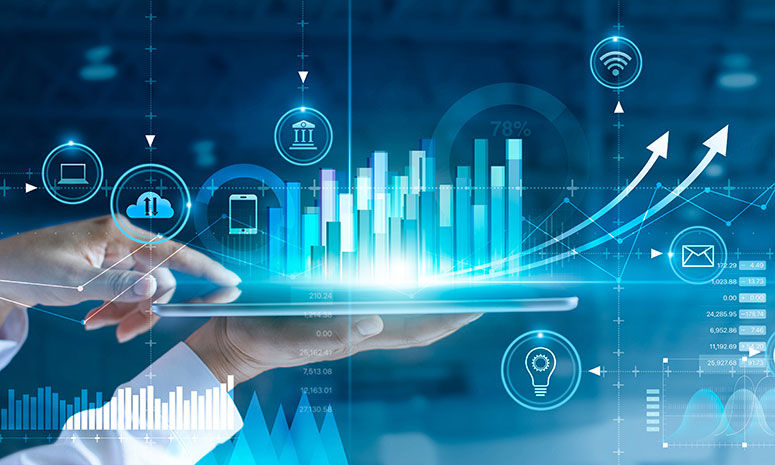
Data Science has rapidly become a cornerstone of modern technology, reshaping industries and influencing decision-making processes. By harnessing the power of vast data sets, data science drives innovation and uncovers valuable insights. This blog explores the development of data science, its core elements, and its significant effects across various fields.
The Evolution of Data Science
Early Beginnings
While data science as a term is relatively recent, its foundations lie in the early days of statistics and data analysis. During the 1960s and 1970s, foundational work in these areas set the stage for what would later become data science. However, the term itself gained prominence in the 2000s with the advent of big data, which demanded more sophisticated analytical techniques.
The Era of Big Data
The rise of big data has profoundly impacted data science. The explosion of data from the internet, social media, and IoT (Internet of Things) has necessitated the development of new technologies and methods for managing and analyzing this vast amount of information. Innovations like Hadoop and Spark have become essential tools in handling big data.
Machine Learning and AI
In recent years, the focus of data science has shifted towards machine learning and artificial intelligence. Machine learning algorithms can detect patterns and make predictions based on historical data, while AI aims to replicate human intelligence. This evolution has expanded the scope of data science, enabling advancements such as personalized recommendations and autonomous systems.
Core Elements of Data Science
Data Collection
The first step in data science is collecting data from diverse sources such as databases, sensors, and online platforms. The quality and extent of this data significantly influence the outcomes of the analysis.
Data Cleaning and Preparation
Data collected often requires thorough cleaning and preparation. This process involves addressing inconsistencies, handling missing values, and transforming data into a format suitable for analysis. Effective data cleaning is critical for ensuring the accuracy and reliability of the results.
Data Analysis
Data analysis involves applying statistical methods and algorithms to interpret the prepared data. This can range from basic descriptive statistics to complex predictive models. Tools like Python, R, and SQL are commonly used during this phase.
Data Visualization
Data visualization is key to interpreting analysis results. By presenting data through charts and graphs, visualization makes complex information more accessible and understandable. Good visualization practices can reveal insights that might not be apparent from raw data alone.
Machine Learning and AI
Machine learning and AI represent advanced aspects of data science. Machine learning algorithms automate decision-making and improve with additional data, while AI encompasses a broader range of technologies designed to mimic human cognitive abilities.
The Impact of Data Science
In Healthcare
Data science has transformed healthcare by enabling predictive analytics, personalized treatments, and efficient resource management. For example, machine learning models can predict disease outbreaks or patient readmissions, supporting more informed healthcare decisions.
In Finance
In the finance sector, data science aids in risk management, fraud detection, and algorithmic trading. Predictive models assess credit risk, and AI systems identify fraudulent transactions in real-time, enhancing financial security.
In Retail
Retailers leverage data science to improve customer experiences through personalized recommendations, targeted marketing, and optimized inventory management. Analyzing customer data helps businesses understand buying behaviors and preferences, leading to more effective strategies.
In Transportation
Data science contributes significantly to transportation, from optimizing routes and managing traffic to advancing autonomous vehicles. Data-driven insights enhance efficiency and safety in transportation systems.
Challenges and Future Directions
Despite its benefits, data science faces challenges such as data privacy issues, the need for skilled professionals, and the complexity of managing large datasets. Looking ahead, advancements in quantum computing and AI algorithms are likely to further evolve the field of data science.
Conclusion
Data science has emerged as a powerful force, revolutionizing industries and enhancing decision-making processes. Its journey from early statistics to advanced AI technologies highlights its growing importance. With the rise of specialized programs, such as the Data Science course in Thane, Mumbai, Navi Mumbai, Delhi, Noida and other cities of India individuals are better equipped to contribute to this evolving field. As data continues to expand, the influence of data science will increase, shaping the future of technology and business.
API Connects is a technology services brand that supports enterprises with data, cloud, API, and AI-driven solutions, including IoT solutions and AI and ML services. Consult our engineers for digital transformation. Visit: https://apiconnects.co.nz/ai-automation-machine-learning/