Unlocking Insights: The Power of Data Analytics in Modern Business
- Purva Yadav
- Jun 28, 2024
- 4 min read
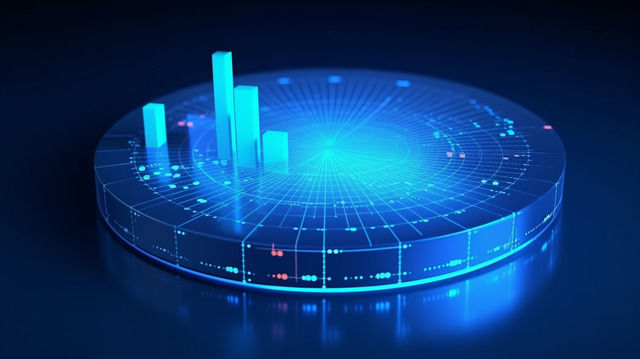
In today's data-driven landscape, data analytics has become essential for businesses seeking a competitive advantage. By converting raw data into valuable insights, data analytics enables companies to make informed decisions, streamline operations, and identify new opportunities. This blog delves into the importance of data analytics, its key components, and its influence across various industries.
Understanding Data Analytics
Analyzing datasets to derive conclusions from the information they contain is known as data analytics. Utilizing techniques ranging from statistical analysis to machine learning, it identifies patterns, correlations, and trends. The primary objective of data analytics is to enhance decision-making and predict future outcomes.
Key Components of Data Analytics
Data Collection and Storage: The backbone of data analytics is high-quality data. Data can be sourced from transaction records, social media interactions, IoT devices, and more. Once collected, data needs to be organized in databases or data warehouses to facilitate analysis.
Data Cleaning and Preprocessing: Inconsistencies and noise are common in raw data. Data cleaning involves removing errors and irrelevant information, while preprocessing formats data for analysis. This step ensures the accuracy and reliability of analytical results.
Exploratory Data Analysis (EDA): EDA involves summarizing the main characteristics of the data, often using visual methods. It helps understand data distribution, identify outliers, and detect underlying patterns. Tools like histograms, scatter plots, and box plots are commonly used in EDA.
Statistical Analysis: Applying statistical techniques to test hypotheses and infer relationships within the data is crucial. Common methods include regression analysis, ANOVA, and chi-square tests, providing a solid foundation for making data-driven decisions.
Machine Learning and Predictive Modeling: Machine learning algorithms are used to build predictive models, which can forecast trends, classify data, and identify patterns not immediately apparent. Techniques such as decision trees, neural networks, and support vector machines are widely used.
Data Visualization: Visual representations of data through charts, graphs, and dashboards help communicate insights effectively. Tools like Tableau, Power BI, and matplotlib make it easy for stakeholders to understand complex data and make quick, informed decisions.
Impact of Data Analytics on Various Industries
Healthcare: Data analytics is transforming healthcare by improving patient outcomes and operational efficiency. Predictive analytics aids in early disease detection and personalized treatment plans, while also optimizing resource allocation and reducing costs.
Finance: In finance, data analytics is vital for risk management, fraud detection, and investment strategies. Predictive models assess credit risk, real-time analytics detect fraudulent transactions, and data analytics helps in portfolio management by identifying profitable investment opportunities.
Retail: Retailers use data analytics to understand customer preferences, optimize pricing strategies, and manage inventory. Analyzing purchase patterns and customer feedback allows for personalized experiences, boosting customer satisfaction and loyalty.
Manufacturing: Data analytics enhances production efficiency and quality control in manufacturing. Predictive maintenance uses sensor data to forecast equipment failures and schedule timely repairs, minimizing downtime. Analytics also streamline supply chain operations, reducing costs and improving delivery times.
Marketing: Marketing strategies are increasingly data-driven. Analyzing consumer behavior and market trends helps businesses design targeted campaigns and measure their effectiveness. Social media analytics track brand sentiment and engagement, providing valuable insights for brand management.
Challenges in Data Analytics
Despite its benefits, data analytics faces several challenges:
Data Privacy and Security: Protecting sensitive data is crucial. Organizations must comply with regulations like GDPR and implement robust security measures to prevent data breaches.
Data Quality: Poor data quality can lead to inaccurate insights and faulty decisions. Investing in data governance practices is essential to maintain data integrity.
Skill Gap: There is a growing demand for skilled data analysts and data scientists. It will cost money to implement training and education initiatives to close this gap.
Integration of Diverse Data Sources: Integrating data from various sources with different formats and structures can be complex. Developing a unified data strategy is necessary for effective analytics.
Future Trends in Data Analytics
The field of data analytics is continuously evolving, with several emerging trends:
Artificial Intelligence (AI) Integration: AI enhances data analytics by processing large datasets and generating deeper insights. AI-driven analytics can automate decision-making processes, making them faster and more accurate.
Real-Time Analytics: The rise of IoT and smart devices is increasing the importance of real-time data analytics. Businesses can make immediate decisions based on current data, improving responsiveness and agility.
Edge Computing: Edge computing processes data closer to its source, reducing latency and bandwidth usage. This is particularly useful for real-time applications, such as autonomous vehicles and smart cities.
Data Democratization: Making data accessible to a wider range of users within an organization is becoming a priority. Self-service analytics tools empower non-technical users to perform their analyses, fostering a data-driven culture.
Conclusion
Data analytics is a powerful tool that can transform businesses by providing actionable insights and enabling informed decision-making. As technology advances, the potential of data analytics continues to grow, offering new opportunities for innovation and efficiency. By embracing data analytics, organizations can stay ahead in a competitive landscape and drive sustainable growth. If you are interested in exploring this further, consider taking a Data Analytics course in Gurgaon, Delhi, Noida, Ghaziabad and other cities of India to deepen your understanding and practical skills in this rapidly evolving field.
Upreports is the fastest-growing white label ORM service in India trusted by entrepreneurs and businesses from all over the globe. It has been rendering personal reputation management services India, pitch deck services in India along with SEO services, social media marketing, reputation management, content creation, and brand-building services for almost 5 years now. Connect with Upreports to discuss your business growth plans. Visit: https://www.upreports.com/white-label-orm-services.html
API Connects is a technology services brand that supports enterprises with data, cloud, API, and AI-driven solutions, including IoT solutions and AI and ML services. Consult our engineers for digital transformation. Visit: https://apiconnects.co.nz/ai-automation-machine-learning/