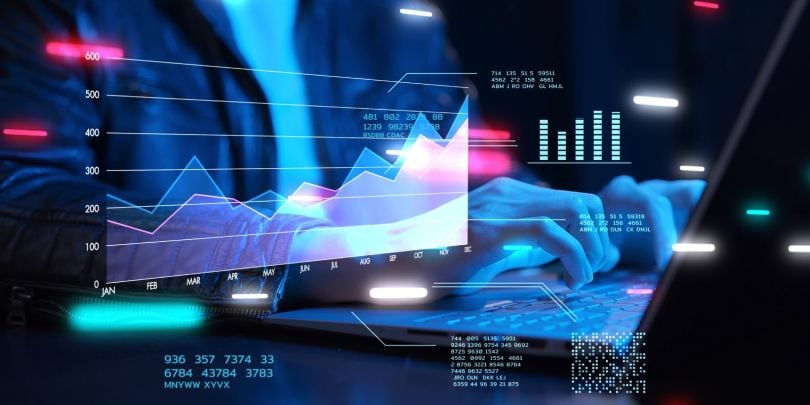
In the digital age, data is omnipresent. From social media activities to online shopping, every digital action generates data. This immense repository of information has the potential to revolutionize industries, drive strategic decisions, and predict future trends. Enter data science, a field that has swiftly become one of the most sought-after careers in the 21st century. But what exactly is data science, and how can one embark on this intriguing journey? Let’s explore.
Understanding Data Science
The multidisciplinary subject of data science uses scientific procedures, systems, algorithms, and methodologies to glean insights and knowledge from both structured and unstructured data. It merges elements of statistics, computer science, and domain-specific knowledge to analyze and interpret complex datasets.
At its essence, data science aims to solve real-world problems by converting raw data into actionable insights. This can involve improving customer experiences, optimizing business operations, predicting market trends, and identifying new opportunities.
Essential Components of Data Science
Data Collection: Gathering data from diverse sources such as databases, web scraping, sensors, and more. This data can be structured (like database tables) or unstructured (like text, images, and videos).
Data Preparation and Cleaning: Inconsistencies, mistakes, and missing numbers are common in raw data. This step involves cleaning and preparing the data to ensure its accuracy and reliability for analysis.
Exploratory Data Analysis (EDA): Summarizing the main characteristics of the data, often through visual methods. EDA helps in understanding the data and uncovering patterns or anomalies.
Model Building: Using statistical methods and machine learning algorithms to create models that can make predictions or classify information based on the data.
Evaluation of the Model: Measuring the Model's Performance using F1 Score, Accuracy, Precision, and Recall. This step ensures the model’s reliability before deployment.
Deployment and Monitoring: Implementing the model in a real-world environment and continuously monitoring its performance to ensure sustained effectiveness.
Tools and Technologies in Data Science
Data scientists utilize various tools and technologies, including:
Programming Languages: Python and R are the most commonly used languages due to their extensive libraries and ease of use.
Data Visualization Tools: Tools like Tableau, Power BI, and Matplotlib help create visual representations of data, facilitating understanding and communication of findings.
Big Data Technologies: Hadoop and Spark are essential for handling large volumes of data that traditional databases cannot manage efficiently.
Machine Learning Frameworks: TensorFlow, Keras, and Scikit-learn are widely used for building and deploying machine learning models.
The Role of a Data Scientist
A data scientist’s role is multifaceted, encompassing responsibilities such as:
Data Analysis: Interpreting complex datasets to identify trends and patterns.
Model Development: Creating algorithms and models to solve specific problems.
Communication: Translating technical findings into actionable business insights that stakeholders can understand.
Problem-Solving: Applying analytical thinking to tackle business challenges and find innovative solutions.
Starting Your Data Science Journey
Learn the Basics: Begin with the fundamentals of statistics, mathematics, and computer science. Online courses, tutorials, and textbooks can provide a strong foundation.
Master Programming Languages: Proficiency in Python or R is crucial, as these languages offer extensive libraries for data manipulation, analysis, and visualization.
Engage in Real Projects: Practical experience is invaluable. Participate in online competitions like Kaggle or work on personal projects that interest you.
Create a Portfolio: Use sites like GitHub to highlight your accomplishments and abilities. A strong portfolio can distinguish you in the job market.
Stay Updated: Data science is a rapidly evolving field. Follow industry blogs, attend webinars, and join professional networks to keep abreast of the latest trends and technologies.
Challenges in Data Science
Despite its many opportunities, data science also presents challenges:
Data Privacy: Ensuring the ethical use of data and compliance with regulations like GDPR.
Data Quality: Dealing with incomplete, noisy, or biased data can affect model accuracy.
Interdisciplinary Nature: The need for knowledge in various domains can be daunting for beginners.
The Future of Data Science
The future of data science is bright, with advancements in artificial intelligence and machine learning propelling the field forward. Innovations in natural language processing, computer vision, and predictive analytics are opening new horizons. As data continues to grow exponentially, the demand for skilled data scientists will only increase, making it a lucrative and exciting career choice.
Conclusion
Starting a journey in data science can be both challenging and rewarding. With a solid grasp of the basics, proficiency in key tools and technologies, and a passion for problem-solving, anyone can harness the power of data. Whether you aim to advance your career or simply explore the world of data, now is the perfect time to dive in and explore the limitless possibilities that data science offers. Enrolling in a Data Science Training Course in Thane, Mumbai, Navi Mumbai, Delhi, Noida and other cities of India can provide the essential knowledge and hands-on experience needed to excel in this dynamic field.
Commenti