Harnessing the Power of Data Analytics: Transforming Insights into Action
- Purva Yadav
- Jun 7, 2024
- 4 min read
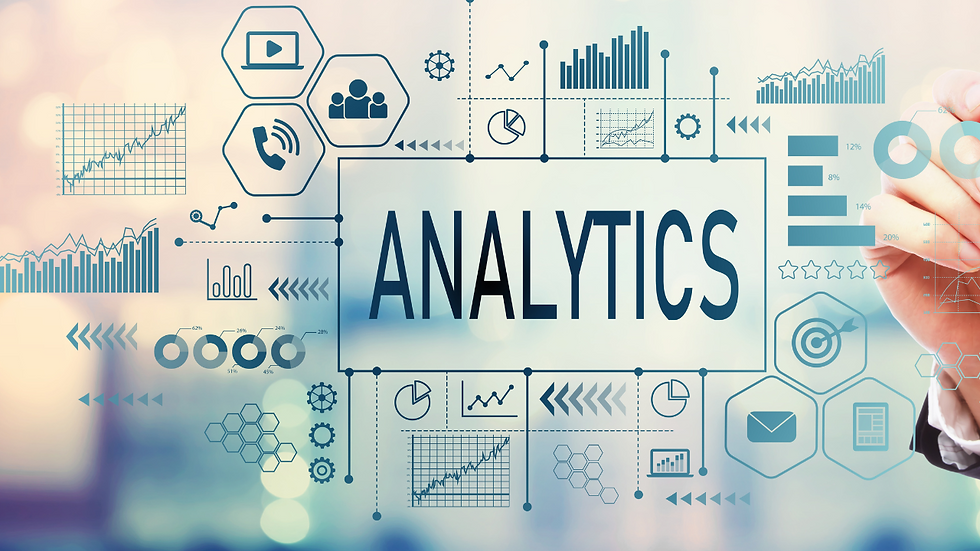
In the digital transformation era, data analytics has become a vital tool for organizations across various sectors. By harnessing vast amounts of data, businesses can identify hidden patterns, gain valuable insights, and make informed decisions that drive growth and innovation. This article delves into the multifaceted realm of data analytics, its methodologies, applications, and the profound impact it has across different industries.
Understanding Data Analytics
Data analytics involves examining raw data to extract meaningful conclusions. It includes several stages: data collection, processing, analysis, and visualization, aiming to turn data into actionable insights for strategic decision-making. There are four main types of data analytics:
Descriptive Analytics: Summarizes historical data to understand past events, using techniques like data aggregation and mining to identify trends and patterns.
Diagnostic Analytics: Explores why something happened by examining data to uncover causal relationships and root causes.
Predictive Analytics: Forecasts future results based on past data using machine learning algorithms and statistical models.
Prescriptive Analytics: The most advanced form, which suggests specific actions to achieve desired outcomes, combining insights from predictive analytics with optimization techniques.
Methodologies in Data Analytics
Data Mining: Extracts valuable information from large datasets using techniques such as classification, clustering, regression, and association rule learning to discover patterns and relationships.
Machine Learning: A subset of AI, where algorithms learn from data and make predictions or decisions without explicit programming, using methods like supervised, unsupervised, and reinforcement learning.
Statistical Analysis: Uses statistical methods to collect, review, analyze, and draw conclusions from data, with techniques including hypothesis testing, ANOVA, regression analysis, and time-series analysis.
Natural Language Processing (NLP): Enables machines to understand and interpret human language, used in text analytics to process unstructured data from sources like social media, reviews, and news articles.
Data Visualization: Represents data graphically through charts, graphs, and maps, aiding in the comprehension of complex data and effective communication of insights.
Applications of Data Analytics
Healthcare: Revolutionizes healthcare by enabling personalized medicine, improving patient outcomes, and optimizing operations. Predictive analytics can forecast disease outbreaks, while prescriptive analytics aids in treatment planning and resource allocation.
Finance: Utilized for risk management, fraud detection, and investment strategies. Predictive models assess credit risk, and machine learning algorithms detect fraudulent transactions in real time.
Retail: Helps retailers understand consumer behavior, optimize inventory, and personalize marketing strategies. Descriptive analytics analyzes sales data, while predictive analytics forecasts demand and trends.
Manufacturing: Enhances processes through predictive maintenance, quality control, and supply chain optimization. Analyzing sensor data predicts equipment failures and reduces downtime.
Marketing: Drives customer segmentation, campaign effectiveness, and ROI analysis. NLP analyzes customer feedback, and machine learning models predict customer churn and recommend personalized offers.
Transportation and Logistics: Improves route optimization, fleet management, and supply chain logistics. Predictive models anticipate delivery delays, and prescriptive analytics optimize shipping routes.
Energy: Utilized for demand forecasting, grid optimization, and predictive maintenance. Analyzing data from smart meters manages energy consumption and reduces costs.
Challenges in Data Analytics
Despite its advantages, data analytics faces several challenges:
Data Quality: Ensuring data accuracy, completeness, and consistency is crucial for reliable analysis. Poor data quality can lead to misleading insights and flawed decisions.
Data Integration: Integrating data from diverse sources like databases, cloud services, and IoT devices poses significant challenges, requiring robust ETL (Extract, Transform, Load) processes and data governance frameworks.
Data Privacy and Security: Protecting data privacy and security is essential, especially with increasing data collection. Compliance with regulations like GDPR and CCPA is crucial for safeguarding sensitive information.
Skill Gap: There's a high demand for skilled data scientists and analysts who can interpret complex data and derive actionable insights. Addressing this skill gap requires investment in education and training programs.
Scalability: As data volumes grow, scalable solutions are needed for efficient data processing and analysis. Cloud computing and distributed systems can help manage large-scale data analytics.
Future Trends in Data Analytics
The future of data analytics is shaped by several emerging trends:
Artificial Intelligence and Machine Learning: AI and ML will continue to advance data analytics. Deep learning techniques and advanced algorithms will enable more accurate predictions and automated decision-making.
Big Data Analytics: The growth of big data will lead to more sophisticated analytics techniques, with real-time analytics and streaming data processing becoming increasingly important.
Edge Analytics: With the rise of IoT devices, edge analytics will gain importance. Analyzing data closer to the source reduces latency and enhances real-time decision-making.
Augmented Analytics: Involves using AI to automate data preparation, insight generation, and explanation, democratizing data analytics by enabling business users to perform complex analyses without deep technical expertise.
Explainable AI: As AI models become more complex, ensuring their transparency and understandability will be crucial for gaining trust and ensuring ethical AI use.
Conclusion
In today's data-driven environment, data analytics has become essential. Organizations can boost productivity, spur innovation, and make well-informed decisions by turning raw data into actionable insights. Despite challenges like data quality, privacy concerns, and skill gaps, the future of data analytics is promising, with advancements in AI, big data, and edge computing paving the way for more sophisticated and accessible analytics solutions. A Data Analytics Training Course in Navi Mumbai, Mumbai, Thane, Noida, Delhi and other cities of India can help bridge the skill gap, equipping professionals with the necessary expertise. Embracing data analytics is not just an option but a necessity for businesses aiming to remain competitive and thrive in the digital age.
Comments