Future of Data Science in Industries & Career Beginnings
- Purva Yadav
- Aug 12, 2024
- 4 min read
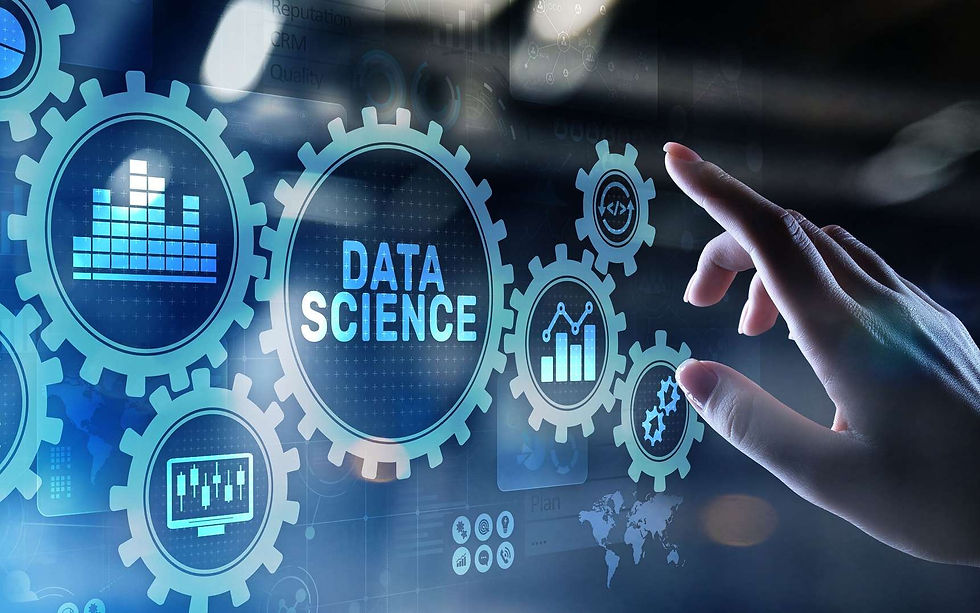
In today’s digital world, data is often considered the new gold. Every click, swipe, and interaction generates vast amounts of information, offering valuable insights into consumer behavior, business operations, and societal trends. But how do we transform this sea of data into meaningful knowledge? The answer lies in data science—a multidisciplinary field that blends statistics, computer science, and domain expertise to convert raw data into actionable insights. This blog will delve into the significance of data science across various industries, the essential skills needed to succeed, and how you can start your journey in this exciting field.
The Impact of Data Science on Modern Industries
Data science has significantly transformed industries across the spectrum. In healthcare, data scientists analyze patient data to enhance treatment outcomes and predict disease outbreaks. In finance, they employ machine learning algorithms to detect fraud and optimize trading strategies. E-commerce companies utilize data science to tailor shopping experiences to individual preferences, while in manufacturing, predictive analytics helps streamline production processes and minimize downtime.
One of the most profound impacts of data science is in decision-making. Traditionally, decisions were often based on intuition or limited data, but data science allows businesses to make informed decisions backed by thorough data analysis. This shift has led to increased efficiency, cost reduction, and improved customer satisfaction.
Core Components of Data Science
Data science is a broad and multifaceted field with several key components:
Data Collection: The first step in any data science project is gathering data, which can involve scraping websites, querying databases, or using sensors in IoT devices. The data collected may be structured, like in databases, or unstructured, such as text or images.
Data Cleaning: Raw data is often messy, with missing values, duplicates, or errors. Data cleaning is a crucial step that ensures the data is accurate and ready for analysis.
Data Analysis: Once the data is cleaned, it’s time to analyze it. This stage may involve statistical analysis, machine learning models, or data visualization techniques to uncover patterns and insights.
Modeling: In many cases, data scientists build predictive models to forecast future trends or classify data. This include choosing suitable methods, using historical data to train the model, and optimizing it for accuracy.
Communication: A data scientist’s role doesn’t end with analysis. They must also communicate their findings to stakeholders through reports, dashboards, or presentations, ensuring that the insights lead to actionable decisions.
Essential Skills for Data Science
Excelling in data science requires a combination of technical and soft skills:
Programming: Proficiency in programming languages like Python or R is essential, as these are used for data manipulation, analysis, and building machine learning models.
Statistics and Mathematics: A solid foundation in statistics and mathematics is crucial for understanding data distributions, probability, and the algorithms that underpin machine learning.
Data Visualization: Tools like Tableau, Power BI, or libraries such as Matplotlib and Seaborn in Python are vital for creating visual representations of data that make insights easier to comprehend.
Machine Learning: Understanding machine learning algorithms, such as linear regression, decision trees, and neural networks, is key to building predictive models.
Domain Knowledge: While technical skills are important, understanding the specific industry you’re working in—whether healthcare, finance, or marketing—enables you to derive more meaningful insights.
Communication: The ability to convey complex ideas clearly and concisely is essential to ensure that your insights translate into action.
Starting Your Data Science Journey
Embarking on a career in data science may seem overwhelming, but with the right approach, it’s entirely achievable. The following actions will help you:
Educational Background: While many data scientists have degrees in fields like computer science, statistics, or engineering, it’s not a strict requirement. Your capacity to comprehend and use data science principles is what counts most. Many successful data scientists have transitioned from unrelated fields.
Online Courses: There are many websites that provide courses in data science. Platforms like Coursera, Uncodemy, edX, and Udacity provide comprehensive programs covering everything from programming to machine learning.
Hands-on Practice: Theory alone isn’t enough; practice is key. Websites like Kaggle offer datasets and competitions where you can apply your skills to real-world problems. Participating in these competitions is a great way to build your portfolio and gain practical experience.
Build Projects: Start with small personal projects, such as analyzing public datasets or creating visualizations. As you gain confidence, take on more complex projects like building predictive models or developing data-driven applications.
Networking: Join data science communities both online and offline. To network with industry experts, go to conferences, webinars, and meetings. Collaborations and employment opportunities can result from networking.
Stay Updated: Data science is a discipline that is always changing. Keep up with the latest trends, tools, and techniques by following blogs, reading research papers, and taking advanced courses.
Career Paths in Data Science
Data scientists are in high demand across a number of businesses. Here are some common career paths:
Data Scientist: This primary role involves analyzing data, building models, and deriving insights to solve business problems.
Data Analyst: Focuses more on analyzing data to create reports and visualizations that assist businesses in decision-making.
Machine Learning Engineer: Specializes in building and deploying machine learning models at scale.
Data Engineer: Works on the infrastructure side, ensuring that data pipelines are efficient and that data is stored correctly for analysis.
Business Analyst: Combines data analysis with business knowledge to provide insights that drive business strategies.
Conclusion
Data science is an essential tool for contemporary organizations and industries, not merely a trendy term. Data science brings up a world of possibilities, whether it is for process optimization, market trend prediction, or customer behavior analysis. In this ever-changing industry, anyone can succeed in the workforce with the appropriate abilities and dedication. The initial step in learning these crucial abilities can be taking part in a data science training course in Thane, Mumbai, Navi Mumbai, Delhi, Noida, or other Indian cities. Data scientists will play an increasingly important role as the world's data output increases, making this a stimulating and fulfilling career path.
Comentários