Exploring Data Analytics: A Comprehensive Guide
- Purva Yadav
- Jun 14, 2024
- 3 min read
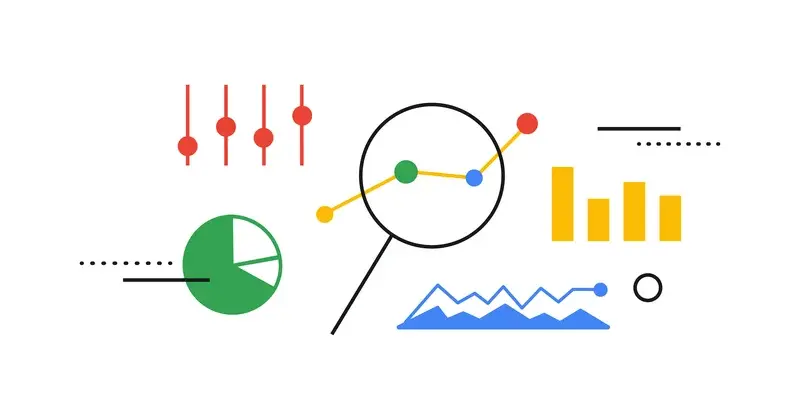
The current digital environment generates data at a never-before-seen rate. Every online interaction, transaction, and social media post leaves a data trail. Analyzing and interpreting this data has become an essential skill for businesses and organizations. Analyzing raw data to find patterns, trends, and insights that can guide decision-making is known as data analytics. This blog will explore the key aspects of data analytics, its significance, techniques, tools, and the future of this dynamic field.
What is Data Analytics?
Analyzing datasets to make inferences about the information they hold is known as data analytics. It involves various techniques and tools to clean, transform, and model data, aiming to discover valuable information, make informed conclusions, and support decision-making. Four primary categories of data analytics exist:
Descriptive Analytics: Summarizes historical data to understand past events, answering the question, "What happened?"
Diagnostic Analytics: Investigates why something happened, delving deeper into data to find causes of events and behaviors.
Predictive Analytics: Forecasts future trends based on historical data, addressing the question, "What could happen?"
Prescriptive Analytics: Offers recommendations for actions based on predictive analytics, answering, "What should we do?"
The Importance of Data Analytics
Data analytics plays a crucial role across various industries for several reasons:
Improved Decision-Making: Data-driven insights enable businesses to make informed decisions rather than relying on intuition.
Increased Efficiency: Identifying patterns and trends helps streamline operations and reduce waste.
Enhanced Customer Experience: Understanding customer behavior allows for personalized marketing and better customer service.
Competitive Advantage: Leveraging data analytics gives companies a competitive edge by better understanding market trends and customer needs.
Key Techniques in Data Analytics
Data analytics encompasses several techniques, each serving a specific purpose. Some commonly used techniques include:
Data Mining: Exploring large datasets to discover patterns and relationships using statistical and machine learning methods to identify trends and anomalies.
Statistical Analysis: Using statistical methods to analyze data, including regression analysis, hypothesis testing, and ANOVA, to understand data distributions and relationships.
Machine Learning: Training algorithms to make predictions or decisions based on data, widely used in predictive analytics for tasks like forecasting and classification.
Text Analysis: Analyzing text data from sources like social media, emails, and reviews for sentiment analysis, topic modeling, and extracting insights from unstructured data.
Visualization: Presenting data in graphical formats, such as dashboards, charts, and graphs, to make it easier to understand and interpret.
Popular Tools for Data Analytics
Several tools are available for data analytics, ranging from programming languages to specialized software. Popular tools include:
Python: Known for its simplicity and versatility, Python is widely used for data analysis and machine learning, with libraries like Pandas, NumPy, and Scikit-learn offering powerful functionalities.
R: Designed for statistical analysis, R has a rich ecosystem of packages for data manipulation, statistical modeling, and visualization.
SQL: Essential for managing and querying relational databases, SQL is used to extract and manipulate data stored in databases.
Tableau: This visualization tool allows users to create interactive and shareable dashboards, enabling quick insights from data.
Excel: Despite being basic, Excel is widely used for data analysis, offering functionalities for data manipulation, statistical analysis, and visualization.
The Future of Data Analytics
The field of data analytics is continuously evolving, driven by technological advancements and increasing data availability. Trends shaping the future of data analytics include:
Artificial Intelligence and Machine Learning: AI and ML are becoming integral to data analytics, enabling more accurate predictions, automation of complex tasks, and deeper insights from data.
Big Data Technologies: As data volumes grow, big data technologies like Hadoop and Spark are essential for efficiently processing and analyzing large datasets.
Real-Time Analytics: Real-time data analysis is becoming crucial for industries like finance, healthcare, and e-commerce, allowing for immediate decision-making and timely responses.
Data Privacy and Security: With increasing focus on data privacy, analytics tools and techniques must comply with regulations like GDPR, ensuring data security and ethical use of data.
Democratization of Data: More organizations are adopting self-service analytics tools, enabling non-technical users to perform data analysis, empowering more people to leverage data for decision-making.
Conclusion
With the use of data analytics, one may effectively convert unstructured data into insightful knowledge. Its applications span across various industries, driving better decision-making, improving efficiency, and enhancing customer experiences. As technology continues to advance, the capabilities and importance of data analytics will only grow. By staying informed about the latest trends and continuously honing analytical skills, such as through a Data Analytics Training Course in Navi Mumbai, Mumbai, Thane, Noida, Delhi and other cities of India, individuals and organizations can harness the full potential of data analytics to achieve their goals. Data analytics is not just about numbers; it's about telling a story with data. By understanding and interpreting data, we can uncover hidden patterns, make informed decisions, and drive progress in a data-driven world.
Comments